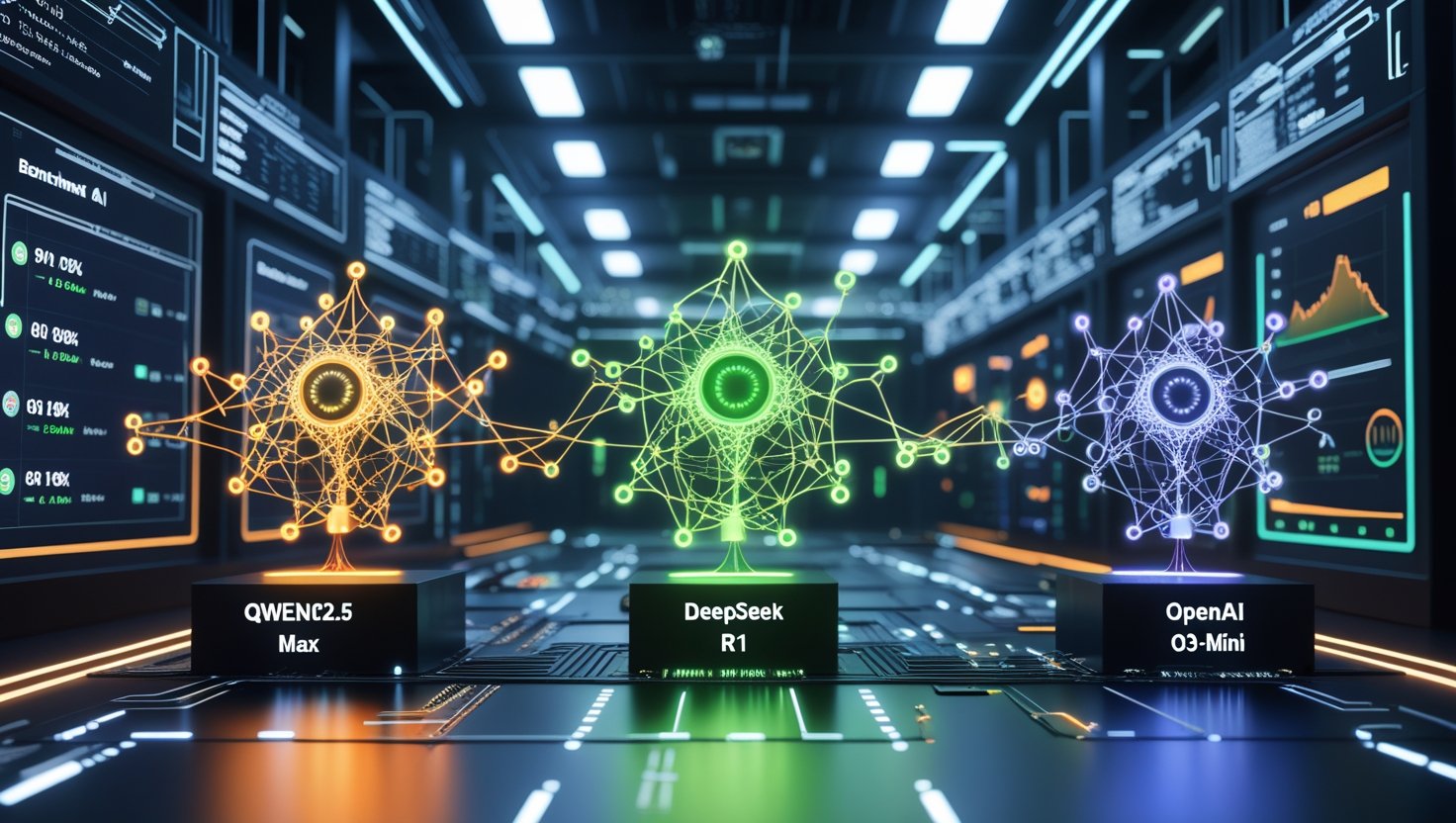
AI Model Showdown: Qwen2.5 Max vs. DeepSeek R1 vs. OpenAI o3-mini – A Technical Comparison
The AI Race of 2025: Diving Deep into Qwen2.5 Max, DeepSeek R1, and OpenAI o3-mini
The AI landscape in early 2025 has seen three major players emerge as leaders in large-scale reasoning models: Alibaba’s Qwen2.5 Max, DeepSeek R1, and OpenAI’s o3-mini. These models are pushing the boundaries of computational intelligence, in different domains, ranging from natural language reasoning to STEM-based problem-solving. The following article is a technical comparison, aiming to break down their respective architectural design, performance benchmarks, and pricing models, to facilitate business and developer decisions.
1. Technical Specifications: Compute, Context, and Training Data
A model's performance is defined by its ability to process massive amounts of computations, context length, and training data diversity. The following are key specifications:
Qwen2.5 Max
Training Data: ~20 Trillion Tokens
Context Window: High (exact details not disclosed)
Compute Requirements: Undisclosed
DeepSeek R1
Training Data: ~13 Trillion Tokens
Context Window: Moderate
Compute Requirements: Undisclosed
o3-mini
Training Data: Not publicly disclosed
Context Window: Adjustable Inference Depth
Compute Requirements: Undisclosed
Takeaways:
Qwen2.5 Max has been trained on the largest dataset and is very efficient for knowledge-intensive tasks.
DeepSeek R1 employs Mixture-of-Experts (MoE) to achieve efficiency at scale.
o3-mini does not report training data size but is optimized for fast, accurate STEM-based applications.
2. Architectural Design and Core Innovations
Each model is designed with a different architecture to maximize its strengths. Let's dive into their basic architectures:
Qwen2.5 Max
Architectural Approach: Transformer-based with enhanced logic & reasoning
Core Strengths: Best for general knowledge and logic-based NLP tasks
DeepSeek R1
Architectural Approach: MoE-based, dynamically activating experts
Core Strengths: Efficient for batch inference & cost optimization
o3-mini
Architectural Approach: Optimized for fast inference & modular adaptability
Core Strengths: Best for STEM, coding, and real-time AI applications
Takeaways
Qwen 2.5 Max Best suited for large enterprises with deep knowledge and reasoning deep into the concepts.
DeepSeek R1 Optimizes big query performance and lowers inference cost.
o3-mini Ideal for developers, engineers, or research institutions for coding and STEM applications.
3. Performance Benchmarks: Measuring AI Strengths
Performance is measured across several standardized benchmarks to measure real-world efficiency:
MMLU (General Knowledge)
Qwen2.5 Max: 85+
DeepSeek R1: ~80
o3-mini: ~75
BBH (Big Bench Hard)
Qwen2.5 Max: 88
DeepSeek R1: 82
o3-mini: 78
C-Eval (Chinese NLP Tasks)
Qwen2.5 Max: 90+
DeepSeek R1: ~85
o3-mini: ~80
Codeforces (Coding Performance)
Qwen2.5 Max: 78
DeepSeek R1: 82
o3-mini: 90+
GSM8K (Math & Logic)
Qwen2.5 Max: 80
DeepSeek R1: 82
o3-mini: 92+
Analysis:
Qwen2.5 Max dominates in general knowledge, logic, and NLP-based tasks.
DeepSeek R1 is a strong competitor, especially in efficiency-driven tasks.
o3-mini is the best performer in STEM and programming, making it an excellent choice for software development.
4. Pricing Model: Cost vs. Performance
Pricing is the first factor when selecting a model. Here are some cost per million tokens in USD:
Qwen2.5 Max
Estimated Cost: ~$0.015 - $0.025 per million tokens
Best For: Enterprises & knowledge-intensive applications
DeepSeek R1
Estimated Cost: ~$0.010 - $0.020 per million tokens
Best For: Efficient large-scale batch inference
o3-mini
Estimated Cost: ~$0.030+ per million tokens
Best For: Premium coding & STEM applications
Takeaways:
Qwen2.5 Max is the best value-for-money, high-quality NLP application.
DeepSeek R1 is an ideal mid-range model that provides a perfect balance between cost and performance.
The no3-mini is premium-priced but justified with its class-leading efficiency for STEM and coding applications.
Conclusion: Which Model to Buy?
Every model has strengths and is therefore suited to a particular use case:
Qwen2.5 Max is a great choice for general knowledge processing, advanced reasoning, and NLP applications in an affordable package.
DeepSeek R1 would be preferred for batch inference efficiency, cost-effectiveness, and MoE-based processing.
You need high-performance STEM reasoning, software development support, and real-time AI adaptability. Choose OpenAI o3-mini.
As AI matures, these models represent the state of the art in terms of reasonability and computational efficiency. Businesses, researchers, and developers must consider their needs and weigh those against the strengths of each model in order to make the right choice.
Keep following Skill Bloomer for the latest updates and advancements in AI!